Oxford Robotics Institute | Robots
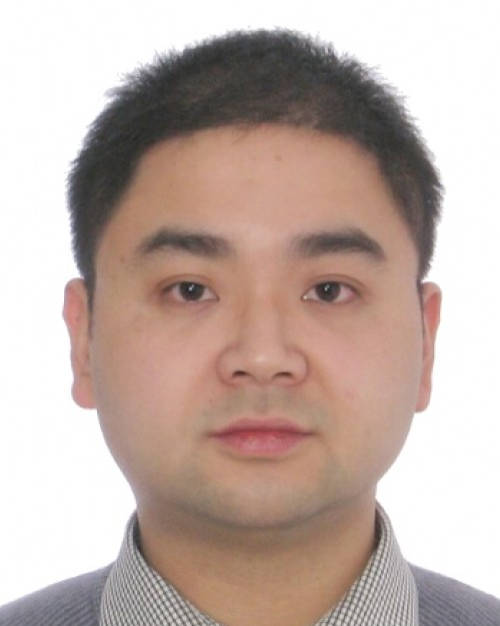
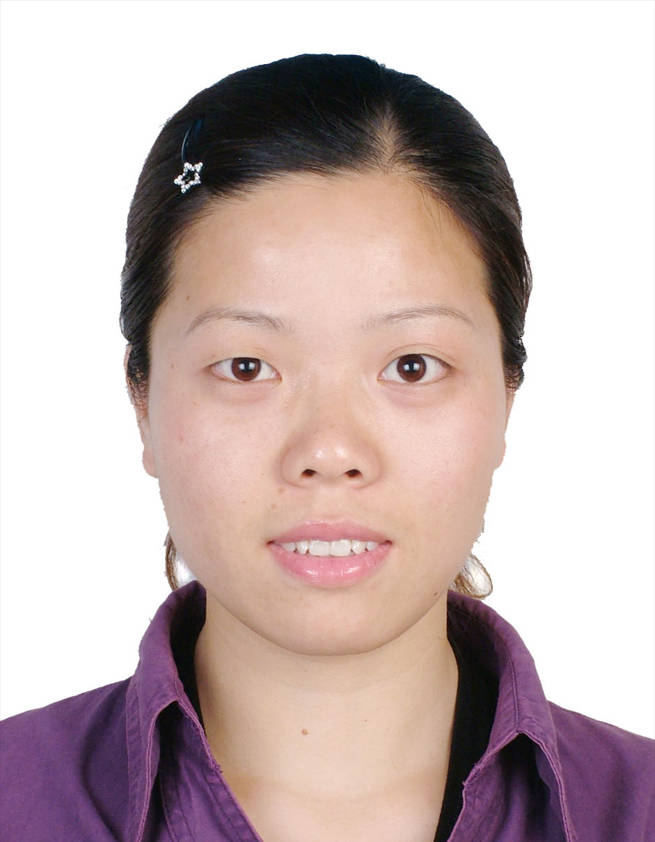
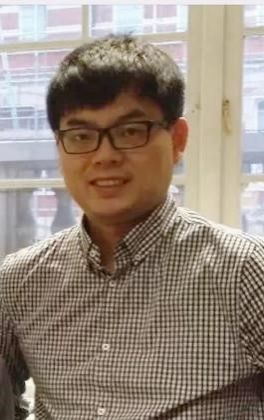
Visual Semantic Fusion
Since November 2023, research has been conducted on scene text detection and recognition methods based on a multimodal framework. To address the issue that current multimodal approaches typically use a single-granularity feature fusion strategy, which fails to fully leverage prior contextual information, a Multi-Granularity Visual Semantic Interactive Fusion Network (MGN-Net) has been proposed. This network includes: (1) a Visual Semantic Multi-Granularity Feature Extraction Network (VSMN); and (2) a Multi-Granularity Graph Fusion Learning Network (MGFN).
Intelligent Interactive Video
Since February 2024, a team of students has been working on the development of an intelligent English learning website based on AI large models. Leveraging cutting-edge AI technology, the intelligent English learning platform we have developed offers a range of powerful features, including intelligent human-machine dialogue, interactive video learning, as well as automatic question generation and automatic scoring. These features provide learners with an easy and efficient learning experience, helping them effectively improve their English skills.
MGFN for Multimodal Scene Text Spotting
Recent scene text spotting methods have shifted to multimodal frameworks, but most rely on single-granularity feature fusion, failing to fully capture the visual-linguistic context. We propose a novel model, Multi-Granularity Visual Semantic Interactive Fusion Network (MGN-Net), which includes a Visual Semantic Multi-Granularity Feature Extraction Network (VSMN) and a Multi-Granularity Graph Fusion Learning Network (MGFN). The VSMN extracts multi-granularity features to enrich contextual relationships, while the MGFN aligns features across modalities and hierarchies for deep fusion. This approach overcomes the limitations of sequential structures in irregular text. Experiments show MGN-Net outperforms existing models. Code will be released.
International competition
In the individual task at ICDAR 2024, we ranked third. The first place in this task was taken by: Bilibili; The second place was taken by: Tianjin University; The third place was taken by us. In another task, we ranked fifth. The official rankings for this task are: First place: Bilibili Second place: American Express Third place: Tianjin University Fourth place: American Express Fifth place: Ours